Have you ever wondered how YouTube's recommendation system works, guiding you through a labyrinth of videos, enticing you to click and explore?
Behind the scenes, there is a complex web of algorithms and data that determine which videos are suggested to you.
But fear not, for in this discussion, we will demystify YouTube's recommendation system and unravel the secrets behind its personalized suggestions.
From user engagement metrics to analyzing behavior patterns, we will delve into the factors that influence video recommendations and empower you to take control of your YouTube experience.
So, let's dive in and uncover the inner workings of this captivating system.
Key Takeaways
- User engagement metrics and algorithmic analysis play a crucial role in shaping suggested content and improving recommendation accuracy.
- Factors influencing video recommendations include popularity, engagement metrics, user feedback, content relevance, metadata, and the quality and freshness of content.
- Algorithmic video suggestions aim to provide personalized and engaging recommendations by considering factors such as a user's viewing history, engagement, popularity, relevance, and similar user behavior.
- User preferences and interests are taken into account by analyzing patterns in user behavior, machine learning algorithms, user feedback, collaborations, and cross-promotion strategies.
The Role of User Engagement Metrics
User engagement metrics play a critical role in YouTube's recommendation system, shaping the content that's suggested to users based on their interactions and preferences. These metrics provide valuable insights into user behavior, allowing YouTube to continuously improve the accuracy of its recommendations.
By analyzing user feedback and monitoring various engagement metrics, YouTube can better understand what types of content users find engaging and relevant. This information is then used to fine-tune the recommendation algorithm, ensuring that users are presented with videos that align with their interests.
One key metric that YouTube considers is recommendation accuracy. This metric measures how well the recommended videos match the users' preferences. YouTube constantly evaluates the performance of its recommendation system by comparing the videos that users interact with and the videos that were recommended to them. By analyzing this data, YouTube can identify patterns and trends, which enables them to make adjustments to the algorithm and improve the accuracy of future recommendations.
User feedback is another important metric that YouTube takes into account. By actively seeking feedback from users, through methods such as surveys or comments, YouTube gains valuable insights into user satisfaction and preferences. This feedback helps YouTube understand how well the recommendations are serving users' needs and whether any changes need to be made.
Understanding YouTube's Algorithm
YouTube's recommendation system relies on a complex algorithm to analyze user engagement metrics and deliver personalized content suggestions. This algorithm takes into account various factors such as watch time, likes, dislikes, comments, and shares to determine the relevance and quality of a video. By analyzing these metrics, YouTube's algorithm aims to understand a user's preferences and interests, enabling it to recommend videos that are likely to be of interest to the user.
However, it's important to consider the ethical implications of YouTube's algorithm. One concern is the potential for the algorithm to create filter bubbles, where users are only exposed to content that aligns with their existing beliefs and opinions. This can lead to the reinforcement of biases and the spread of misinformation. Additionally, YouTube's algorithm is designed to maximize user engagement and watch time, which can incentivize the recommendation of clickbait or sensationalist content. This raises questions about the impact of the algorithm on the quality and diversity of content on the platform.
Moreover, the monetization aspect of YouTube's recommendation system also raises ethical concerns. The algorithm prioritizes videos that are likely to generate ad revenue, potentially favoring creators who produce content that appeals to advertisers rather than content that's informative or valuable to users.
Factors Influencing Video Recommendations
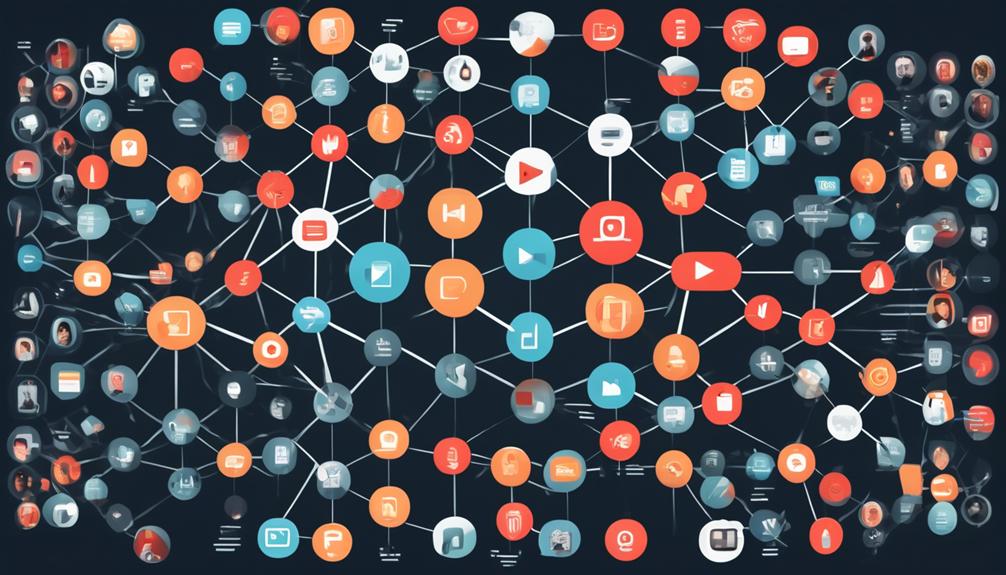
The factors that influence YouTube's video recommendations can be categorized into three main areas.
First, the algorithmic video suggestions take into account various data points such as video popularity, engagement metrics, and user feedback.
Second, user viewing behavior, including watch history, likes, and subscriptions, plays a significant role in shaping the recommendations.
Finally, content relevance factors like video title, description, and tags are considered to ensure that the recommended videos align with the user's interests and preferences.
These factors work together to provide personalized and relevant video recommendations to users on YouTube.
Algorithmic Video Suggestions
Factors influencing video recommendations on YouTube's algorithmic system can significantly impact the content that users are exposed to. YouTube employs algorithmic filtering and recommender systems to determine which videos to suggest to users. These algorithms take into account various factors to make recommendations that are tailored to each user's preferences and interests.
One important factor is the user's viewing history, as the algorithm analyzes the videos they've previously watched to understand their preferences. Additionally, the algorithm considers factors such as user engagement, video popularity, and relevance to the user's search queries. The algorithm also looks at the behavior of similar users and the overall viewing patterns on the platform.
Through these intricate mechanisms, YouTube's recommendation system aims to provide users with a personalized and engaging video-watching experience.
User Viewing Behavior
Understanding user viewing behavior is crucial in determining the factors that influence video recommendations on YouTube's algorithmic system. Through user engagement analysis, YouTube gathers valuable data on how users interact with videos. This analysis includes factors such as the duration of video views, likes, comments, and shares.
By analyzing these user behaviors, YouTube's recommendation system can optimize video suggestions to cater to individual preferences. For example, if a user frequently watches videos on cooking, the algorithm will prioritize recommending cooking-related content. This fine-tuning of recommendations based on user viewing behavior enhances the overall user experience by providing personalized and relevant content.
Furthermore, recommendation system optimization ensures that users continue to engage with the platform, leading to increased watch time and user satisfaction.
Content Relevance Factors
Building upon the analysis of user viewing behavior, the YouTube recommendation system takes into account various content relevance factors to determine the most suitable video recommendations for each user. These factors play a crucial role in enhancing user interaction and improving content discovery on the platform.
One important content relevance factor is the metadata associated with a video. This includes the title, description, tags, and category, which provide information about the video's topic, genre, and keywords. By analyzing this metadata, the recommendation system can understand the content of the video and match it with relevant user interests.
Another factor is the engagement level of a video. The recommendation system takes into consideration metrics such as views, likes, comments, and shares to gauge the popularity and user reception of a video. Videos that have higher engagement are more likely to be recommended to users who've shown similar interests or viewing patterns.
Furthermore, the recommendation system considers the quality and freshness of the content. It takes into account factors such as video resolution, production value, and upload date. Higher quality videos are more likely to be recommended as they provide a better viewing experience.
Analyzing User Behavior Patterns
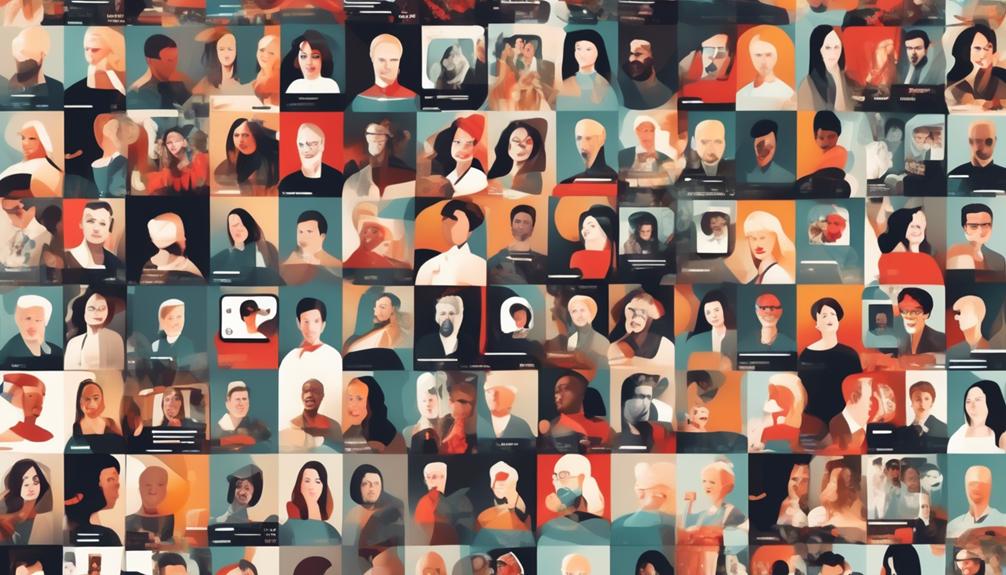
By analyzing user behavior patterns, YouTube's recommendation system gains valuable insights into user preferences and interests. This analysis involves examining click through rates (CTRs) and conducting user retention analysis. CTRs measure the number of times a user clicks on recommended videos, indicating their level of interest in the content. YouTube's recommendation system analyzes CTRs to determine the effectiveness of its recommendations and to identify patterns in user behavior. By understanding which recommendations are most likely to be clicked on, the system can improve its suggestions and provide users with more relevant content.
User retention analysis is another crucial aspect of understanding user behavior patterns. It involves analyzing how long users stay on a video after clicking on it. By examining this metric, YouTube can determine if a recommended video is engaging enough to hold users' attention. If users consistently click away from a particular video shortly after starting it, it suggests that the recommendation wasn't relevant or appealing to the user. This data helps YouTube refine its recommendation algorithm and deliver more engaging and personalized content.
Analyzing user behavior patterns is essential for YouTube's recommendation system to continuously improve. By understanding user preferences and interests through CTR analysis and user retention analysis, YouTube can provide more accurate and relevant recommendations, enhancing the user experience and increasing user satisfaction.
The Impact of Watch History and Search Queries
When it comes to YouTube's recommendation system, your watch history and search queries play a significant role. By analyzing your past viewing habits and the keywords you use to search for content, YouTube is able to understand your preferences and provide personalized video suggestions.
This algorithmic impact on content ensures that the recommendations you receive align with your interests, ultimately enhancing your overall viewing experience.
User Preferences and Recommendations
The impact of your watch history and search queries on YouTube's recommendation system is crucial in shaping the personalized content you encounter on the platform. YouTube's recommendation system takes into account your past viewing habits and search queries to understand your preferences and interests. By analyzing this data, the system can generate recommendations that align with your tastes and desires.
User feedback plays a significant role in fine-tuning these recommendations. YouTube employs machine learning algorithms to process vast amounts of data and identify patterns in user behavior. These algorithms continuously learn and adapt based on your interactions with the platform. They analyze factors such as the types of videos you watch, the duration of your viewing sessions, and the frequency of your searches to deliver content that's most likely to engage and satisfy you.
Personalized Video Suggestions
Your watch history and search queries on YouTube have a significant impact on the personalized video suggestions you receive. YouTube's recommendation system relies on complex filtering algorithms to analyze your viewing behavior and search queries to curate a tailored selection of videos that align with your interests. These algorithms take into account various factors, such as the types of videos you have watched, the duration of your viewing sessions, and the frequency of your searches.
By analyzing this data, YouTube's recommendation system aims to surface videos that are more likely to engage and entertain you. Additionally, user feedback analysis plays a crucial role in refining the personalized video suggestions. YouTube takes into consideration feedback, such as likes, dislikes, and comments, to further improve the accuracy of its recommendations.
Through this iterative process of analyzing user data and feedback, YouTube continually strives to enhance the quality of its personalized video suggestions.
Algorithmic Impact on Content
The impact of your watch history and search queries on YouTube's recommendation system is reflected in the algorithmic curation of personalized video suggestions. YouTube's recommendation algorithm takes into account your previous viewing habits and search queries to predict your interests and preferences. This algorithmic impact on content raises important questions regarding user privacy and ethical implications.
On one hand, the personalized video suggestions enhance user experience by offering relevant and engaging content. By analyzing your watch history and search queries, YouTube can provide a personalized feed tailored to your interests, saving you time and effort in searching for videos that align with your preferences.
However, this algorithmic curation also raises concerns about user privacy. The collection and analysis of user data, including watch history and search queries, can potentially infringe on user privacy rights. The ethical implications of this algorithmic impact on content warrant careful consideration, as it highlights the delicate balance between providing personalized recommendations and respecting user privacy.
Collaborations and Cross-Promotion Strategies
Collaborations and cross-promotion strategies play a crucial role in optimizing YouTube's recommendation system. These strategies, such as cross-platform collaborations and influencer marketing strategies, are designed to increase visibility and engagement for content creators.
By partnering with other creators or brands, YouTubers can tap into new audiences and expand their reach.
Cross-platform collaborations involve working with creators from different social media platforms, such as Instagram or TikTok. This allows creators to leverage their existing fan base and introduce their content to a wider audience. By collaborating with creators who have a similar target audience, YouTubers can gain more exposure and potentially attract new subscribers.
Influencer marketing strategies involve partnering with brands to promote their products or services. This type of collaboration often includes sponsored content, where YouTubers integrate the brand's offerings into their videos. By doing so, creators can monetize their content while also providing their audience with valuable information about the brand.
These collaborations and cross-promotion strategies not only benefit individual creators but also contribute to the overall effectiveness of YouTube's recommendation system. When YouTube's algorithm detects that a video is receiving high engagement and viewership due to a collaboration, it may recommend that video to a broader audience. This, in turn, increases the visibility of both the collaborating creators and the platform as a whole.
How YouTube Personalizes Recommendations
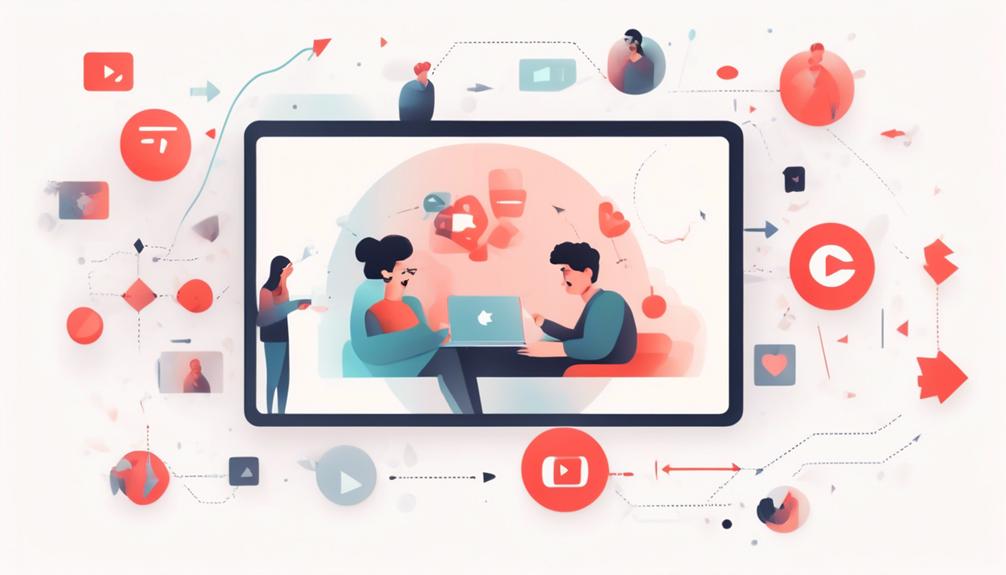
Optimizing YouTube's recommendation system involves understanding how the platform personalizes recommendations based on user preferences and viewing history. To achieve this, YouTube utilizes machine learning techniques to analyze vast amounts of data and provide users with personalized content suggestions.
User feedback plays a crucial role in personalizing recommendations on YouTube. The platform collects explicit feedback from users, such as likes, dislikes, and comments, to understand their preferences better. This information helps YouTube to refine its recommendation algorithms and deliver content that aligns with each user's individual interests.
In addition to explicit feedback, YouTube also considers implicit feedback, which is derived from user behavior and viewing history. By analyzing the videos users watch, the duration of their viewing sessions, and the frequency of their interactions, YouTube gains insights into their preferences and tailors recommendations accordingly.
Machine learning techniques are at the heart of YouTube's recommendation system. These algorithms process the vast amount of user data collected and learn patterns and trends to make predictions about each user's preferences. By continuously analyzing and adapting to user interactions, YouTube's recommendation system evolves over time, becoming more accurate and personalized.
Taking Control of Your YouTube Recommendations
To customize your YouTube recommendations and take control of your viewing experience, you can adjust your settings and preferences. YouTube provides various tools and options that allow you to manage and control the content that's recommended to you. By understanding how to control your recommendations, you can ensure that YouTube suggests videos that align with your interests and preferences.
One way to control your recommendations is by utilizing the 'Not Interested' feature. When you come across a video that you don't want to see in your recommendations again, you can click on the three-dot menu next to the video and select 'Not Interested.' This action informs YouTube that you aren't interested in that particular video or similar content, helping to improve the accuracy of future recommendations.
Another option is to use the 'Don't Recommend Channel' feature. If you find that a specific channel's content doesn't appeal to you, you can choose to stop receiving recommendations from that channel. Simply click on the three-dot menu on any video from the channel, select 'Don't Recommend Channel,' and YouTube will adjust your recommendations accordingly.
Furthermore, YouTube allows you to control recommendations based on specific topics. You can access these settings by clicking on your profile picture, selecting 'Settings,' and then navigating to the 'Recommendations' tab. Here, you can choose to remove specific topics from your recommendations or indicate topics that you prefer to see more of.
Taking control of your YouTube recommendations empowers you to curate a personalized viewing experience. By utilizing the available tools and adjusting your settings and preferences, you can ensure that YouTube suggests videos that align with your interests and preferences.
Frequently Asked Questions
How Does Youtube Determine the Order in Which Videos Are Recommended on the Homepage?
YouTube determines the order of recommended videos on the homepage based on various factors. User behavior plays a significant role in YouTube's recommendation algorithm, influencing which videos are shown to you.
Can Youtubers Manipulate the Recommendation System to Promote Their Own Videos?
YouTubers can manipulate the recommendation system to promote their own videos, but it raises ethical concerns. The effects of recommendation manipulation on user experience can be detrimental, as it affects the fairness and diversity of content.
What Impact Does the Number of Likes and Dislikes on a Video Have on Its Recommendation?
The number of likes and dislikes on a video significantly impacts its recommendation. Viewer feedback plays a crucial role in YouTube's recommendation algorithm, as it considers engagement to determine which videos to suggest to users.
Does Youtube Prioritize Promoting Videos From Popular Channels Over Smaller Ones?
Does YouTube prioritize promoting videos from popular channels over smaller ones? Yes, YouTube's promoting algorithm takes into account various factors, such as engagement metrics and user preferences, to determine content visibility, which can favor popular channels.
How Does the Recommendation System Account for Different Languages and Regions?
YouTube's recommendation system takes into account regional diversity and language preferences to provide you with relevant content. By analyzing your viewing history and demographics, it tailors recommendations to your language and region, ensuring a personalized experience.
Conclusion
In conclusion, understanding YouTube's recommendation system is crucial for content creators and users alike.
One interesting statistic that highlights the system's impact is that 70% of watch time on YouTube is driven by the recommended videos. This statistic emphasizes the significant role that recommendations play in shaping user behavior and highlights the importance of optimizing content to align with the algorithm.
By analyzing user behavior patterns and leveraging collaborations, content creators can maximize their reach and engagement on the platform.